Visual Perception via Learning in an Open World
The 3rd workshop on Open World Vision
Location: West 118-120
Time: 9:00am - 17:15pm Vancouver Local Time (PDT), June 18, 2023
The recorded workshop is released on YouTube: https://youtu.be/TUG3IBU5JFo
in conjunction with CVPR 2023, Vancouver, Canada
Overview
Visual perception is indispensable for numerous applications, spanning transportation, healthcare, security, commerce, entertainment, and interdisciplinary research. Visual perception algorithms developed in a closed-world setup often generalize poorly to the real open-world, which contains situations that are never-before-seen, dynamic, vast, and unpredictable.
This requires visual perception algorithms to be developed for the open-world, to address its complexities such as recognizing unknown objects, debiasing imbalanced data distributions, leveraging multimodal signals, efficient few-shot learning, etc. Moreover, today's most powerful visual perception models are pretrained in an open-world, e.g., training them on web-scale data consisting of images, langauges and so on. We are in the best era to study Visual Perception via Learning in an Open World (VPLOW). Therefore, we are inviting you to our VPLOW workshop, where multiple speakers and challenge competitions will cover a variety of topics of VPLOW. We hope our workshop stimulates fruitful discussions.
You might be interested in our previous workshops:
Topics
Topics of interest include, but are not limited to:
- data: long-tailed distribution, open-set, unknowns, streaming data, biased data, unlabeled data, anomaly, multi-modality, etc.
- concepts: open-vocabulary, ontology/taxonomy of object classes, evolving class ontology, etc.
- learning: X-shot learning, Y-supervised learning, lifelong/continual learning, domain adaptation/generalization, open-world learning, multimodal pretraining, prompt learning, foundation model tuning, etc.
- social impact: safety, fairness, real-world applications, inter-disciplinary research, etc.
- misc: datasets, benchmarks, interpretability, robustness, generalization, etc.
Examples
Let's consider the following motivational examples.
- Open-world data follows a long-tail distribution. Data tends to follow a long-tailed distribution and real-world tasks often emphasize the rarely-seen data. A model trained on such long-tailed data can perform poorly on rare or underrepresentative data. For example, a visual recognition model can misclassify underrepresented minorities and make unethical predictions (ref. case1, case2).
- Open-world contains unknown examples. Largely due to the long-tail nature of data distribution, visual perception models are invariably confronted by unknown examples in the open world. Failing to detecting the unknowns can cause serious issues. For example, a Tesla Model 3 did not identify an unknown overturned truck and crashed into the truck (ref. case).
- Open-world requires learning with evolving data, and labels. The world of interest is changing over time, e.g., driving scenes (in different cities and under different weather), the search engine ("apple" means different things today and 20 years ago). This says that the data distribution and semantics are continually changing and evolving. How to address distribution shifts and concept drifts?
Speakers
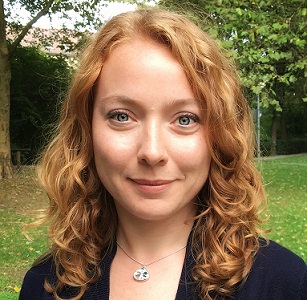
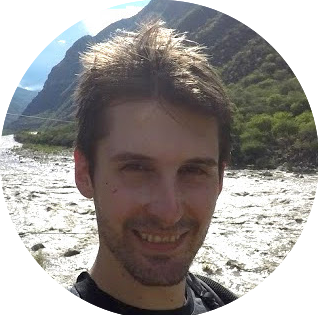